Today people now regard analytics as one of the most progressive and useful sciences in the 21st century. It defines the process of studying data and making something out of it to deduce something from the details, set policies, and make discoveries. As a result, there is a stronghold of machine learning in data science.
Machine Learning (ML) is a subfield of AI that helps the computer build predictions or make decisions by feeding data without programming the application for each assignment. It is also necessary to note that the combination of Machine Learning and Data Science practiced during the last two decades has become an integral part of business, government, and organizational decision-making, problem-solving, optimization, and innovation.
What is Data Science?
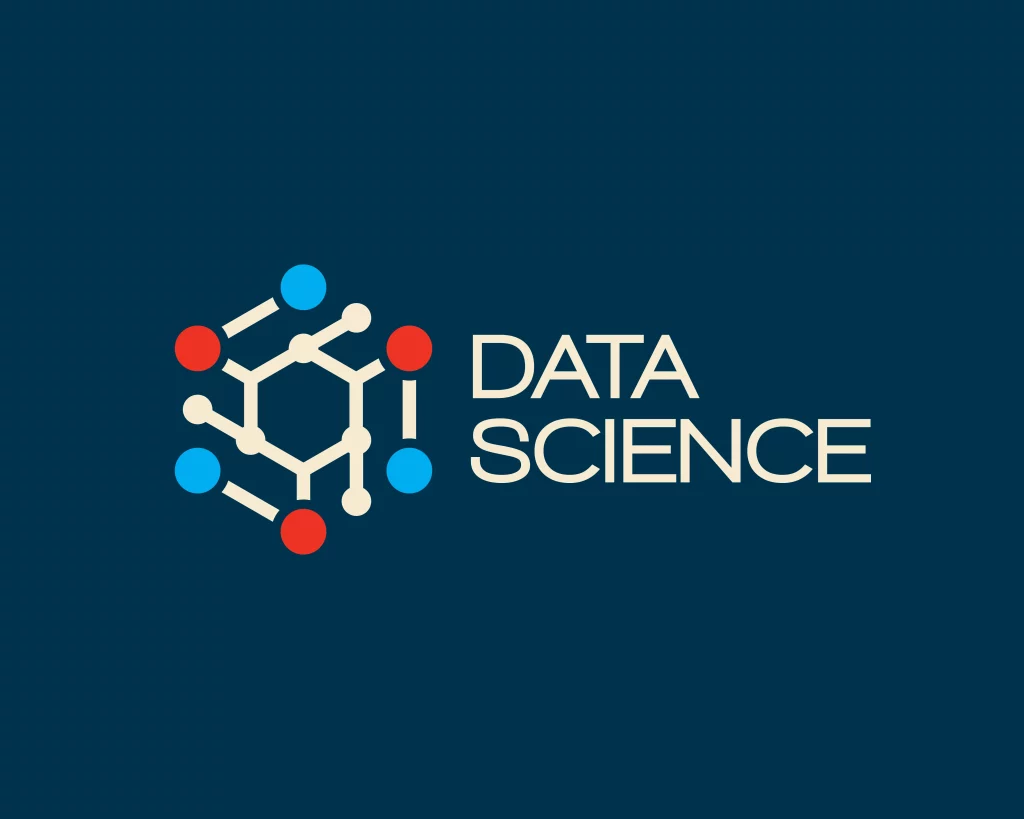
Data science brings together several fields, including math, statistics, computer science, and specific subject knowledge. The explosion of information from social media, sensors, business systems, etc. requires new ways to handle this flood of data. The past problems of handling data were unsuitable for managing the large volumes, speed, and diversification of today’s big data. Therefore, this need led to the development of data science as a new discipline. Data Science is driven by data scientists henceforth, data scientists and machine learning go hand in hand as they are the people responsible for turning raw data into meaningful insights.
What is Machine Learning?
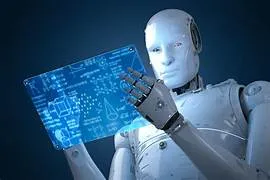
Artificial intelligence or AI comprising machine learning is a method of data analysis that automatically builds analytical models. It is for the assumption that systems are capable of learning from the data and making decisions on their own. AI as a field itself started in the Twentieth century and the real progress of machine learning was made in the 1990s and 2000s due to improvements in technology, the power of computational devices, and the presence of Big Data. There are many impacts of machine learning in data science in terms of functioning with sophisticated characteristics in data as well as the potential for consideration proportionally by large datasets. Data Scientists have used these to take on problems largely considered interactable when approached with classical statistical methods alone.
An Insight on the Development of Machine Learning in Data Science
Machine learning has been so successful in data science, mainly because of a variety of techniques available to play with and figure out the patterns that can be broadly divided into three different areas which are Supervised Learning, Unsupervised Learning, and Reinforcement Learning. All of these methodologies are built for specific scenarios and operations, as well as various kinds of data.
- Supervised Learning: Data Scientists mostly use supervised learning when it comes to machine learning in data science. This consists of training a model on a labeled dataset, having both the input and the corresponding output. For example, the model will learn a function that maps inputs to outputs, and can then use this mapping to predict new input data. Supervised learning is good for classification and regression tasks.
- Unsupervised Learning: Unsupervised learning performs on unlabelled data, in which it has to discover some hidden patterns or intrinsic structures from the data without needing guidance. It is particularly beneficial for the phase of exploratory data analysis, where there is no specific outcome expected. SMOTE implements an algorithm that specifies how to group similar data points. Some of the famous clustering algorithms are k-means, hierarchical clustering, and DBSCAN. People commonly use it in applications such as market segmentation, social network analysis, and customer behavior modeling.
- Reinforcement Learning: One of the important categories of ML is Reinforcement learning (RL). This is an agent learning to solve an environment and make optimum choices to gain maximum of incentives. Reinforcement learning is about learning machine learning in data science through interaction and therefore, observing the results of the performed actions. For instance, RL has been very successful in robotics and has produced strong results in game playing. Its usage is expanding in the realm of data science and machine learning. This can be seen in optimization problems, dynamic pricing models, and real-time recommendation engines.
Real-World Uses of Machine Learning in Data Science
There is no question that machine learning has changed the development of multiple industries by providing data scientists a way to solve too many and too complex data. Here are a few important types of machine learning applications in data science within different areas:
1. Healthcare Industry
One of the classic examples of machine learning in data science is in healthcare- from predictive modeling to diagnostics, and personalized medicine. AI generated about 80% of what you wrote. To predict when diseases may break out, detect patients at high risk and prepare individual treatment regimes this technology goes through patient’s records, lab test results as well as genetic information. For example, researchers mostly use convolutional neural networks (CNNs) in tumor detection or disease classification tasks.
2. Finance Sector
Many institutions in the financial industry have already started employing data scientists and machine learning for purposes, including fraud detection, credit scoring, or algorithmic trading. For transactional data which can be indicative of fraud, supervised learning models such as random forests and gradient boosting machines are frequently employed to detect any anomalies Machine learning is used to optimize portfolios, predict stock prices, and manage the risk.
3. Retail and E-commerce Industry
The retail industry is one of the sectors that has benefited from machine learning the most, as it has yielded more accurate recommendation systems, customer segmentation, and demand forecasting. Amazon employs a content-based recommendation algorithm and collaborative filtering to recommend products, goods, and services targeted at users based on the preferences of their typical past behaviors whereas Netflix on the other hand suggests new shows or movies that users should watch built up from prior interests. Another instance of machine learning in data science is the optimization of the supply chain with the aid of machine learning models that can predict future demand, and optimize inventory cost as well as delivery times.
Kodakco Data Science Course
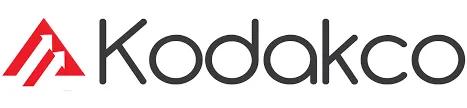
Kodakco is one of the leading players in the field of upskilling and technology consultations. Out of their many courses, the Data Science courses with Python and R are the most recognized ones. The course designers structured the course to reflect the new trends and also Industry-relevant skills.
Standout aspects of Courses provided by Kodakco
- Learn Python and R programming at your own pace
- Receive Certification in data science in Python and R, after course completion
- Postgraduate degree in Data Science
- MBA in Data Science
- Comprehensive Curriculum with flexibility in learning
- Provides Internship as well as Placement support
Conclusion
To sum up, Data science and machine learning have brought about a revolution in several industry sectors including healthcare, finance, and retail among others. This venture provides advanced means to analyze data, make judgments, and refine operations thus positively impacting these areas. however, despite the strides made, a few challenges persist such as model understanding about data quality and ethical concerns. There are very optimistic prospects for the future of machine learning within the field of data science.
Recommended Reads
- Maximizing Your Learning Potential with a Data Science Course
- Top 5 Data Science Masters Programs of 2024
- Top 20 Data Science Courses in India with Placement
FAQs
Ans: Data science combines math, stats, computer science, and field-specific knowledge into one area of study.
Ans: Machine learning in data science has a big effect on healthcare, finance, and retail. It helps analyze data, make choices, and make processes better.
Ans: Machine learning matters because it handles big tricky datasets. This allows for predicting outcomes, doing tasks on its own, and making smart choices based on what the data shows.
Ans: Some tough spots include making sure data is good helping people understand models better, dealing with complex math, and tackling ethical issues like being fair, keeping things private, and staying secure.
Ans: Big changes coming to data science are AutoML to make things easier, AI that explains itself for clarity, and Edge Computing to process stuff right away.